MSU UURAF 2021
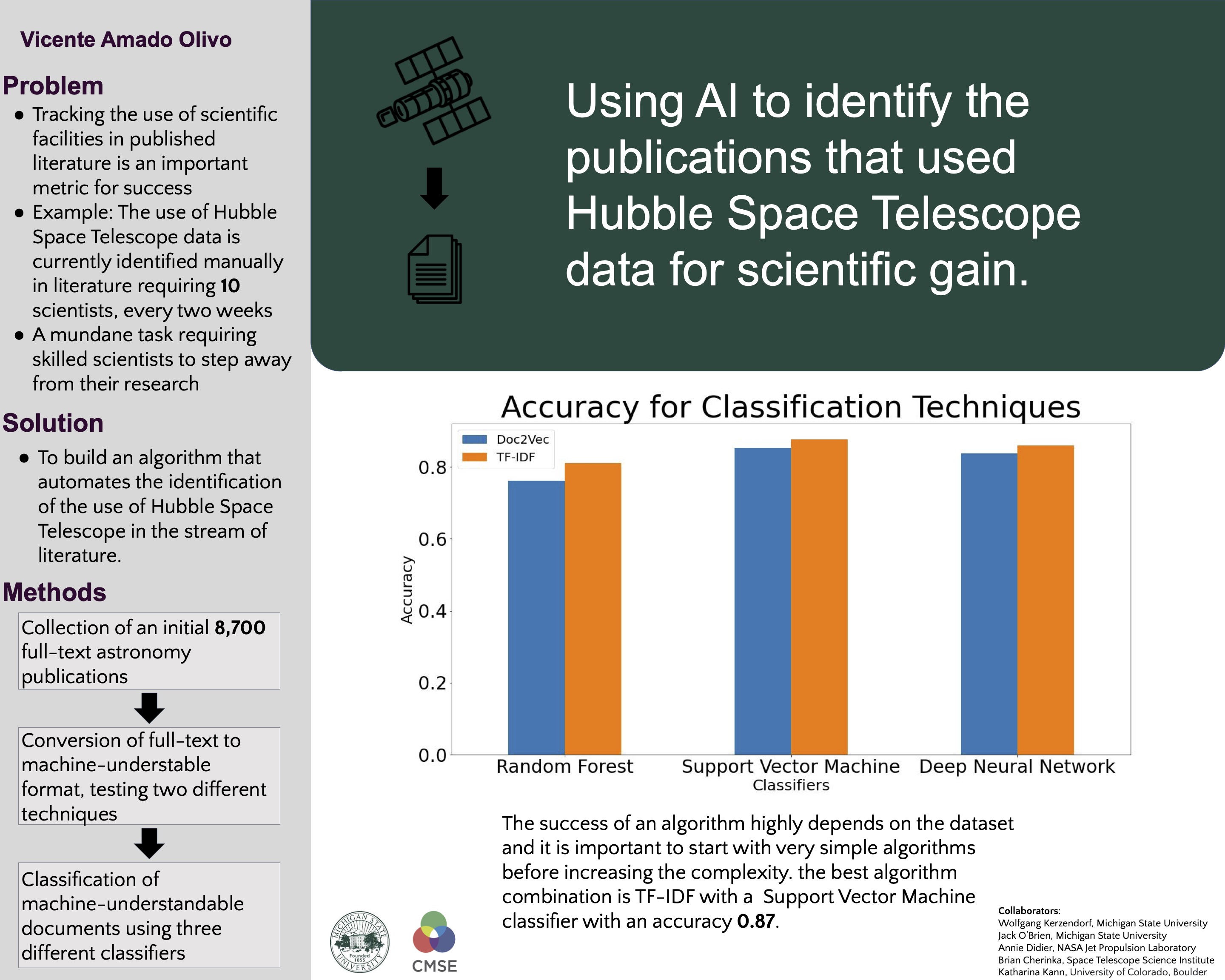
Abstract: The modern scientific community relies on instruments and data provided by large scientific institutions, such as the Hubble Space Telescope and the Large Hadron Collider. For these institutions to optimally serve the scientific community, it is crucial to track how often and in what way their instruments are being used. The community, however, does not provide usage data directly but embeds this in plain language in their publications. Simple solutions to extract usage information from publications (such as full-text searches) fail as authors often just mention institutions (e.g. Hubble Space Telescope is often mentioned in astronomy papers) even though they do not use them in the presented work. Additionally, the ever-expanding literature introduces new challenges. For example, in astronomy, the number of papers published doubles every 14 years. The current approach is to use the valuable time of scientists at these institutions on the menial task to perform manual searches through the thousands of papers published every year. In this poster, I will present a solution using Natural Language Processing to automatically extract such information with high accuracy from published works. Our algorithm performs with an 87% accuracy on an initial project for the Hubble Space Telescope team that is currently being implemented in the production workflow. One of the main findings of this study is that a focused approach on only parts of the text (paragraphs) performs better than full-text algorithms. I will conclude by providing an outlook on our future work that focuses on using recently published Natural Language Processing algorithms to increase the accuracy of the algorithm.